In recent years, Python has consistently ranked as the most popular programming language according to the TIOBE Index. Its success is driven by several key factors, including its simplicity, versatility, and cross-platform compatibility. Today, it is widely used to develop a wide range of web and mobile applications.
Another important reason for Python’s widespread adoption is its extensive use in artificial intelligence and machine learning development. In this article, we’ll explore Python's role in AI development and explain why it's an excellent choice for building AI-powered applications.
Benefits of Python for AI solutions
The choice of Python for AI projects is driven by the properties of this programming language that facilitate not only the development process but also further modernization and enhancement of the built apps. Here are the most important things that you need to know about Python if you consider it for your AI products.
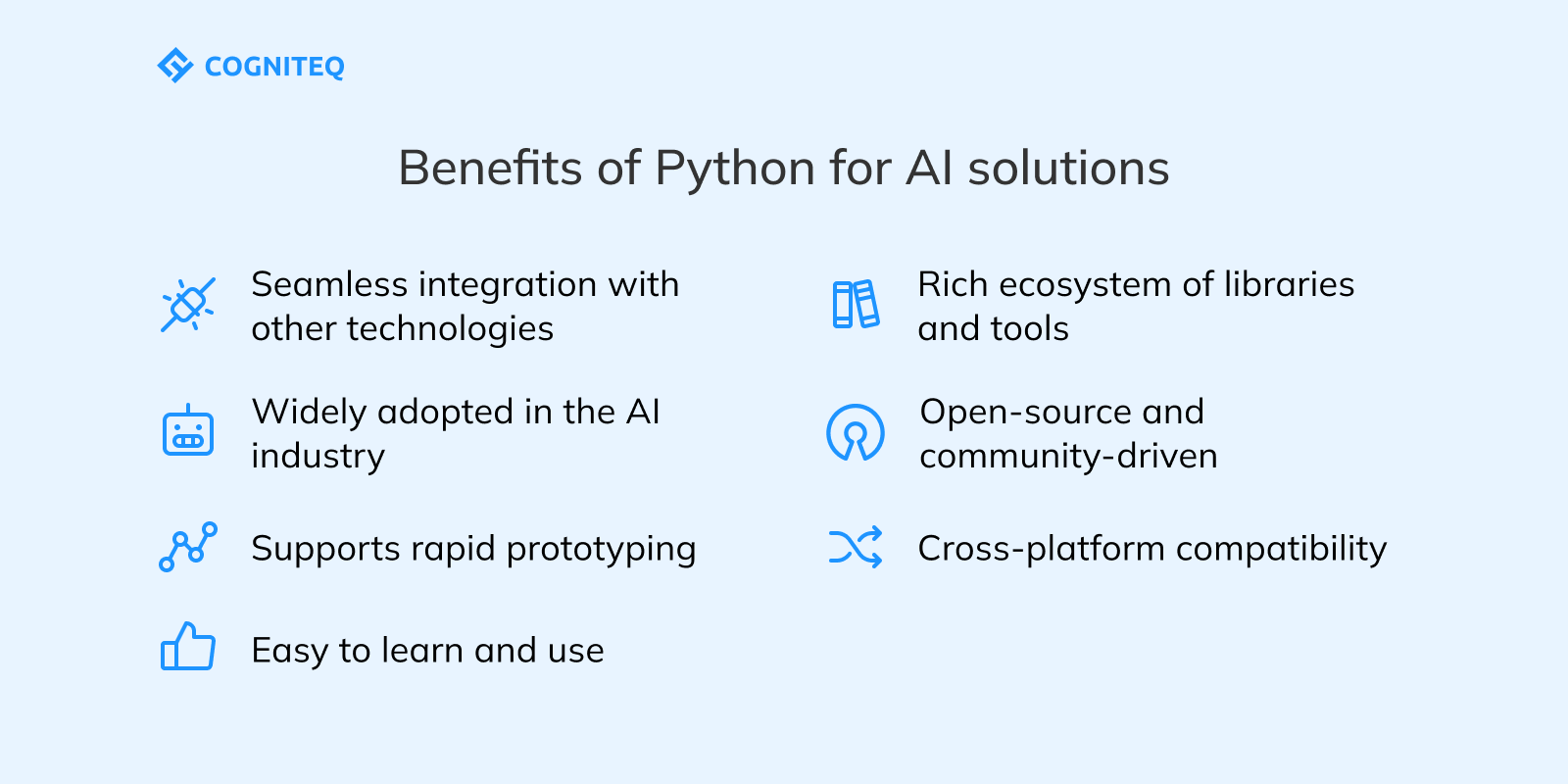
- It is easy to learn and use. Python has a simple, readable syntax. This makes it accessible for beginners and efficient for experienced developers. That’s why this language is quite inclusive for development projects. Moreover, thanks to its straightforward structure, teams can focus on solving AI problems rather than dealing with complex coding.
- Python has a rich ecosystem. Developers who work with Python can leverage a wide choice of libraries and frameworks specifically designed for AI and ML tasks. It’s also vital to highlight the availability of pre-trained AI models in the Python libraries. By using ready-made components and models instead of writing entire code from scratch, it is possible to significantly reduce development time. We will consider some of the popular libraries in more detail further in this blog post.
- It supports rapid prototyping. Python’s simplicity and extensive libraries enable quick prototyping and experimentation. These possibilities are essential for AI development where iterative testing and refinement are commonly applied.
- Python is cross-platform. It means that code written in this programming language can run on various operating systems, such as Windows, macOS, and Linux, without modification. This flexibility is crucial for AI development because quite often models need to be deployed across different environments.
- It seamlessly integrates with other languages and technologies. For instance, this programming language can be easily integrated with C, C++, Java, and .NET; big data tools like Hadoop and Apache Spark; as well as cloud services and APIs. This capability greatly enhances the development of big, scalable AI systems.
- Python is open-source. This programming language is free to use and distribute. As a result, the entrance barrier for individuals and organizations who want to start working with it is rather low. The open-source nature also often becomes a booster for collaboration and innovation within the AI community.
- Python is already widely adopted in the AI industry. Today this programming language has become a standard tool for research and development in AI. Many companies, including tech giants like Google and Meta, use Python for AI projects. This factor is also continuously strengthening its position as a leading language for AI.
Python AI development: Popular libraries
As indicated above, the availability of a rich ecosystem of libraries and frameworks can be a strong argument for many teams to select Python for AI projects.
Python AI libraries are collections of modules and tools that provide pre-defined functions, classes, and methods for building AI apps. With their help, developers can create, train, and deploy AI models without writing all algorithms on their own.
Let’s consider the most widely applied libraries intended for AI programming with Python.
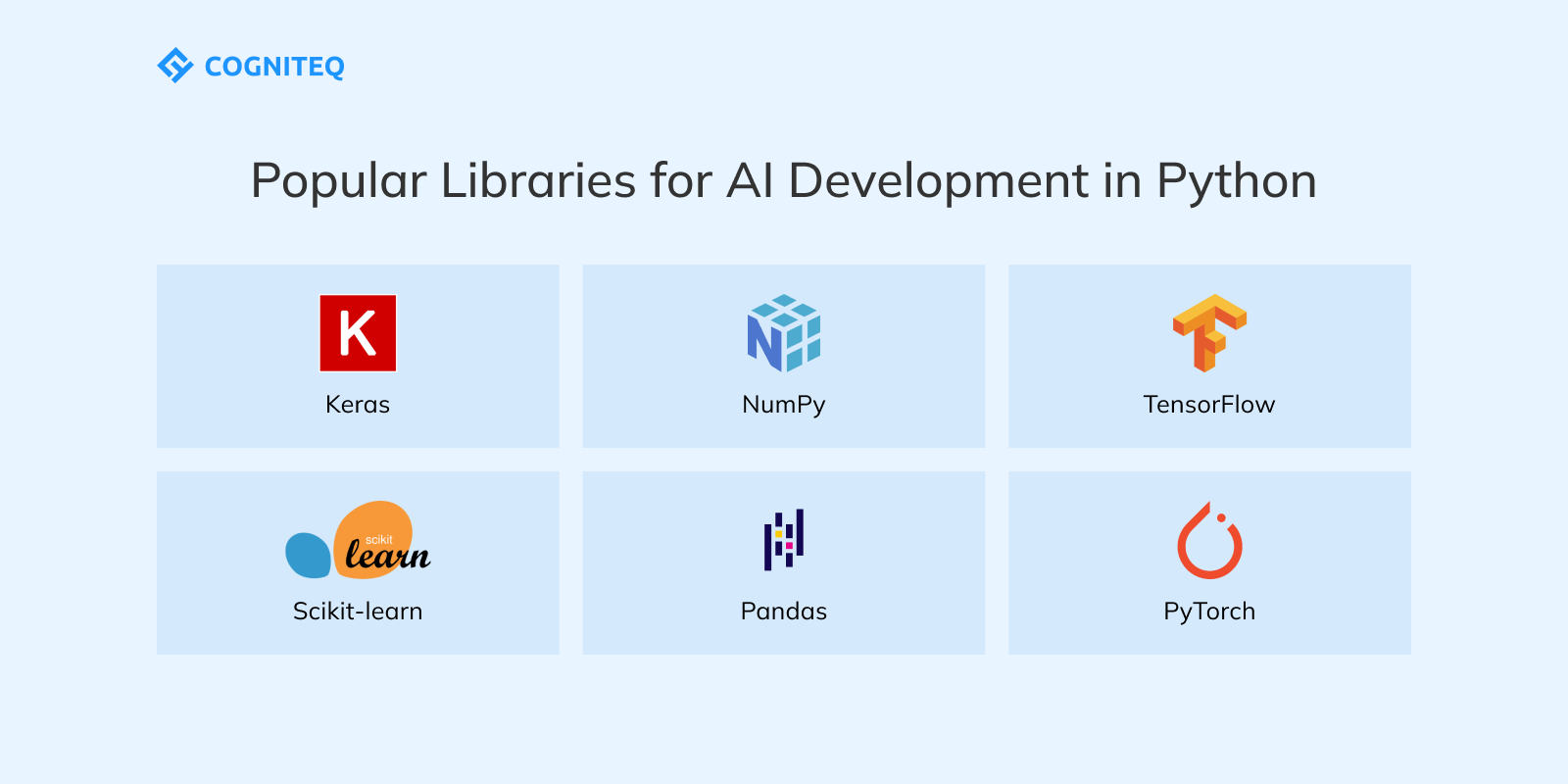
TensorFlow
It is an open-source Python AI library developed by Google. This free-to-use library is designed for building and training machine learning and deep learning models.
TensorFlow is known for its versatility. It can be applied for creating models across various domains, including natural language processing, image and handwriting recognition, and various computational simulations.
Its key advantages include efficient execution of low-level operations on multiple acceleration platforms, scalability for production environments, and seamless graph exportation for interoperability.
The use of TensorFlow doesn’t require any specific expertise. As a result, even those developers who are just learning how to code AI in Python can rely on it.
PyTorch
This dynamic deep learning framework was initially developed by Meta AI (Facebook) but now it is a part of the Linux Foundation family.
Written in C++ and Python, it was released in 2016 as an alternative to its predecessor dubbed Torch. The popularity of the newly introduced framework was growing quickly thanks to the ease of use in building ML models. Another feature that was positively welcomed by developers was the possibility to optimize models at runtime. This makes it highly flexible and intuitive for research.
Today, PyTorch is often chosen for academic purposes and rapid prototyping, as well as specific production use cases.
Scikit-learn
It is a comprehensive open-source library for traditional machine learning algorithms like regression, classification, and clustering. It provides tools for data preprocessing, model evaluation, and hyperparameter tuning.
Scikit-learn offers a uniform API across various models, which enables seamless transitions between algorithms without requiring new syntax. Moreover, it provides a variety of tuning parameters and grid search tools to optimize models for improved performance.
Its ease of use and versatility make it an excellent choice for both beginners and experienced data scientists who need to build and implement machine learning models.
Keras
It is an open-source library that offers a Python interface for developing artificial neural networks. Initially, it was released as independent software. But later, it was integrated into the TensorFlow ecosystem. Today it is seamlessly integrated with other Python AI libraries as well.
One of the key things that developers traditionally like about Keras is modularity. They can work with and efficiently integrate simpler building units while creating complex neural systems. This approach supports code reusability and also simplifies troubleshooting and debugging.
Moreover, the library is designed for speed and efficiency. This allows developers to train even large and complex models within a reasonable timeframe. It also offers tools for model evaluation and performance optimization.
NumPy
That’s another open-source library on our list. It is intended for numerical computing in Python.
NumPy supports large, multi-dimensional arrays and matrices, as well as a wide range of functions for mathematical operations on these arrays. Today, it is commonly used in scientific computing, data analysis, machine learning, and other fields that demand fast and efficient array processing.
NumPy can smoothly interact with many other Python libraries. This facilitates the performance of advanced data visualization tasks and computations.
Pandas
It is a popular library for data manipulation and data analysis. It offers a robust framework for efficiently handling large datasets. Built on top of NumPy, it ensures fast and optimized numerical operations.
Pandas addresses many challenges that data scientists face in conducting research. In data science, handling data typically involves multiple stages, such as data cleaning, analysis, modeling, and structuring results for visualization or tabular representation. Pandas facilitates these and many other essential data-related tasks.
With all its benefits, it has gained mass adoption within the data science community. Therefore, developers can easily find various helpful resources and tutorials, as well as support through dedicated online groups and forums.
Python for AI: What solutions can be built with it?
These days, Python is viewed as a go-to language for building different types of AI solutions intended for different purposes. If you have any ideas for a project powered by artificial intelligence or machine learning in Python, it will be useful for you to have a closer look at the possibilities of this language. Let’s focus on the most popular use cases.
NLP apps
NLP tools play an important role in speech recognition. For example, speech-to-text features convert spoken language into text, while text-to-speech, and vice versa, transform the text into spoken words. Such tools can contribute to the inclusivity of many solutions and services by making them available to people with disabilities. But also, they are often targeted at a wide audience and can provide fully hand-free experiences in interaction with various devices.
Moreover, NLP apps can help to generate text, classify text into categories, translate text between languages, extract various data, recognize individuals based on their voice, as well as detect emotions or sentiments.
Chatbots and virtual assistants
Python is widely used to create conversational AI systems. They can understand user input and respond to user queries in a human-like manner.
Python and its libraries were applied in the development of such popular solutions from this category as ChatGPT, Alexa, and Google Assistant, among others.
Recommendation systems
Recommendation systems help to predict user preferences and suggest items like movies, products, and songs based on historical data, user behavior, or particular item characteristics. Python offers a variety of libraries and frameworks to build recommendation systems efficiently. That’s why it is used in numerous systems of this type across different domains, including eCommerce, social media, entertainment, etc.
For example, such popular services as Spotify and Netflix relied on Python for AI recommendation systems.
Computer vision
Python can be used for building a wide range of computer vision-driven apps. For example, it is possible to create image classification functionality to identify objects or patterns in images. Such tools can be helpful for facial recognition and analysis of medical images.
Object detection features are valuable for self-driving cars and surveillance systems. At the same time, video analysis is used for motion detection, activity recognition, or object tracking.
Looking for a reliable Python AI development team?
At Cogniteq, we have robust expertise in this field and will be able to offer the best approach to your project implementation!
Future of AI programming with Python
Technology is constantly advancing. As new breakthroughs and research emerge in the field of artificial intelligence, the demands and expectations for AI solutions will also shift. To enable developers to meet industry requirements, the technologies used in creating such solutions must adapt accordingly.
How will Python evolve? What changes will we observe already in the near future? Let’s try to predict.
- Ecosystem growth. It is expected that the ecosystem of Python AI libraries will continue to grow. It’s highly likely that we will see new more specialized tools for emerging fields like quantum machine learning and edge AI.
- Democratization of AI. Right now the development of AI solutions requires highly specific skills and knowledge, which seriously limits the circle of experts who can contribute to such projects. With the further adoption of low-code AI platforms, AI will become more accessible to non-experts. Moreover, as the availability of pre-trained models is projected to grow as well, teams will have the possibility to avoid the need for extensive training.
- Focus on stability and performance. While at the moment the key focus is placed on the functionality of AI systems and their accuracy, in the future, it will be shifted more to performance. AI models grow larger. Given this, Python will evolve to handle massive datasets and computations more efficiently.
- Explainable AI (XAI). At the moment, one of the key challenges of using AI models is the lack of transparency. In most cases, in most cases, users can’t know why this or that decision is made. As a result, the efficiency of AI systems for some domains is fully lost. Explainable AI can change the situation. It is expected that a number of Python libraries will evolve to offer more advanced tools for interpreting and explaining AI models.
- AI at the edge. This concept refers to the deployment of AI models directly on edge devices, such as smartphones, IoT devices, sensors, and embedded systems, instead of relying on centralized cloud servers.
This approach brings computation and data processing closer to the source of data generation. As a result, it enables real-time decision-making, reduced latency, and improved privacy. Python will play a crucial role in the mass adoption of AI at the edge and its ecosystem may expand with new lightweight frameworks.
Wrapping up
Python’s simplicity, versatility, and robust ecosystem make it a highly suitable language for developing cutting-edge AI solutions. It has already gained an excellent reputation as a key language for AI development. And at the moment, there aren’t any signs indicating that it is going to lose ground to any other programming languages.
At Cogniteq, we have been working with Python for many years already. We deeply understand all its peculiarities and strengths. This allows us to build scalable and high-performance solutions tailored to the needs of different business domains.
Want to learn more about our services and expertise? Do not hesitate to contact us!
FAQ
What are the reasons for the popularity of Python AI development?
Python stands out for a range of features that are highly valuable for building powerful AI solutions. Among these features, we should mention its simplicity, strong community support, flexibility, and efficient prototyping capabilities. All this makes it a good choice for building machine learning, deep learning, and data science applications for various domains.
Can Python be used for machine learning?
Yes, Python is widely used for the development of ML solutions. It is possible thanks to its rich ecosystem of libraries like TensorFlow, PyTorch, Scikit-learn, and Keras. Apart from this, Python is highly scalable and can be integrated with other languages, including C, C++, and Java. This makes it suitable for large-scale ML applications.
Can Python support NLP tasks?
Today, Python is widely used for natural language processing. To handle various tasks of this type, Python relies on the available libraries. For example, TextBlob can be used for simple NLP tasks like sentiment analysis and text classification. Gensim is helpful for topic modeling and word embeddings. Meanwhile, spaCY is intended for advanced natural language processing, including dependency parsing and named entity recognition.
Is it possible to use Python for building large-scale AI projects?
Python is well-suited for building large-scale AI projects mainly due to its flexibility, scalability, and robust ecosystem. Apart from this, Python can easily integrate with other languages and technologies. This is crucial for large-scale projects that may require combining different systems and tools. Moreover, Python is cross-platform. This is highly beneficial for large teams that may be using different development environments.
Is Python a better choice for AI development than other languages?
Python is widely considered to be one of the best choices for AI solutions. It is well-suited for rapid prototyping, data analysis, and integration with other technologies. At the same time, some other languages like R or Java also have their niches where they demonstrate excellent results. R is good at statistical analysis and data visualization, while Java can be used for large-scale, performance-critical AI applications.